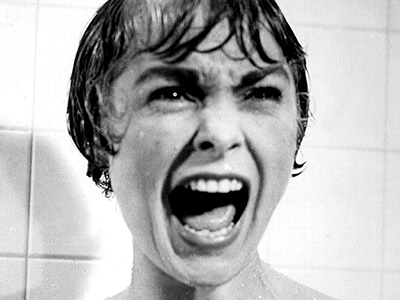
Takeaways
My first roadblock was the limitation of the VADER lexicon. Horror doesn’t translate well with common sentiments of what “good” means. In the time constraints of this project, I chose to remove versions of”horror” and “scare” from the VADER lexicon.
Also, comparing the character counts between subreddits showed a pattern. I assumed initially that my analysis would lead me to refer Kari to a specialty site like r/HorrorMoviesOnly. But the character counts visually displayed how wildly subjective their site was. Therefore, I recommended r/AllThingsHorror because there was a higher possibility of neutral or positive comments instead of negative.